Catalysts are inevitable in the modern-day chemical industry. They are known to speed up any chemical reaction, ultimately demanding reduced equipment size and faster production rates. Most chemical reactions produce multiple products, and we are not usually interested in all of them. Hence, the chemical processing conditions, such as temperature and pressure, must be maintained so that the reaction is directed towards the desired product. The catalyst also helps steer the reaction towards the desired product(s), aside from increasing the overall rate. In the context of CCUS, catalysts are required in converting CO2 into value-added products. For instance, in the process of producing methane from CO2, we use nickel-based catalysts while we use copper-based catalysts for producing methanol.
Now, the question is: How do we select catalysts and design better catalytic reaction systems?
How do we determine the best possible one from an array of catalyst candidates for my given process? How do we know at which temperature and pressure would we get the maximum yield of my desired product? How can I explore better materials for the same process? How do I add appropriate promoters to the same catalyst?
About a century back, the answer to all these questions would have been: “Let’s try out different things in the lab and check”. Discovering the suitable catalyst for the infamous Ammonia synthesis reaction that saved the world and for which Fritz Haber was awarded the Nobel prize also involved many trials and errors. But today, we have a rational approach to answering all these questions. In other words, we have a more affluent and sophisticated understanding of chemical reactions that facilitates the “rational” approach to catalyst and process design.
Let us try to understand this with an example: Assume that we have to reach a specific destination from our place in a given time. We might have to travel through different roads starting from our location. Some roads may be wide, a few might be crowded, and others may be under repair. It is easier for us to travel through the wider roads, whereas if the streets are filled, or under repair, it might take additional time and effort to cross them. Suppose we identify several routes that we could take. In that case, we could analyze them and determine the best routes and the issues with the other routes, like traffic jams or potholes, that may be addressed through various interventions.
This is analogous to producing the desired product at the desired rate. The reactant (which is us being present at our source) might have to travel through different paths to produce the product (which is us reaching the destination). The reaction pathway analysis (RPA) provides us with information through mathematical simulation where we can identify the routes the reactant takes to reach the product. Once we have this data, this can be used to analyze which paths are difficult (i.e., where higher energy inputs are needed) to fix the issues. This helps us gain considerable insight into the reaction system and provide a means for catalyst selection and efficient reactor design to overcome the difficulties.
Although writing “once we fix the issue” was effortless, it is not easy in an absolute sense. Performing the reaction pathway analysis itself involves a lot of complex quantum mechanics calculations that demand severe computational and human effort. Each of the “roads” or the reaction steps in the pathway needs to be quantified – i.e., reaction rate parameters have to be determined for them, which is quite challenging and time-consuming.
Let us say A and B react to form C and D in the presence of a catalyst, where C is the desired product and D is undesired. The reaction pathway for this reaction might look like something, as shown below (assuming we have done all the complex calculations needed for the reaction pathway analysis). It looks like this because A and B get converted to C and D through several intermediate steps, where A*, B*, AB1*, AB2*, C* and D* are the intermediate species through which this overall conversion takes place.
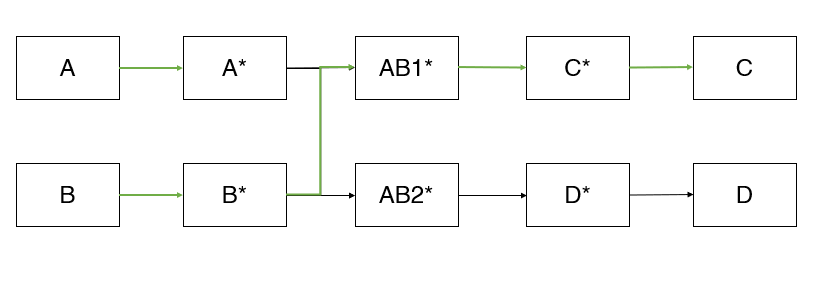
From the pathway, it is evident that A* and B* (* indicates that the species is adsorbed on the catalyst) can react to form either AB1* or AB2*. If A* and B* form AB1*, we will ultimately get the desired product, C. If they react to form AB2*, it will form the undesired product D*. The preferable pathway for our process is shown in green colour. Hence, our original problem has now broken down to a small command: Make sure that A* and B* react to form AB1* and not AB2*.
To do that, we will now return to our original question: How do we find a suitable catalyst for this system? What should be my catalyst so that there is a maximized production of AB1*, that in turn leads to C?
The answer is as follows: When we do rich and sophisticated quantum mechanical calculations, we understand a lot about these species and intermediates. We understand the chemical nature of A*, B*, AB1*, AB2* and so on, which helps us identify the appropriate catalyst. We have a comprehensive database of the property of several materials thanks to all the research that has been done through all these years. This property database of the vast array of materials, along with our chemical intuition, can be used to identify the suitable material that would take us through the “green-coloured” pathway. There could even be many tricky cases where one of the reactions in the green path is “blocked”. Even in these cases, we will know the root cause of the problem (it is like knowing why there is a traffic jam or construction activity on one of the roads). We can resolve this by using a catalyst promoter or tweaking reaction conditions such as temperature and pressure (like sending police officials to clear the jam or directing the traffic better).
Once we have the best-performing catalyst, we need to study their performance in enormous real-time industrial-scale reactors. This translation is not straightforward as industrial-scale reactors usually contain larger particles and their behaviour changes abruptly as compared to lab-scale reactors which comprise relatively smaller particles. As of date, the industrial reactors are designed using existing correlations derived from lab-scale experiments which are neither accurate nor physically meaningful. To visualize the actual scenario inside the reactor under operando conditions and to optimize it, advanced simulations are required. These simulations have the capability to provide local and detailed information and are exceptionally accurate. However, these simulations consume a lot of computational time, even for modelling lab-scale reactors.
As one could easily understand from this article, the simulations cover a wide range of time and length scales, from atomistic to a reactor scale, rightly called “multiscale modelling”, and this proves to be a handy tool in catalyst discovery and reactor optimization. The image below schematically explains multiscale modelling with length and timescale descriptions required for catalytic reactions.
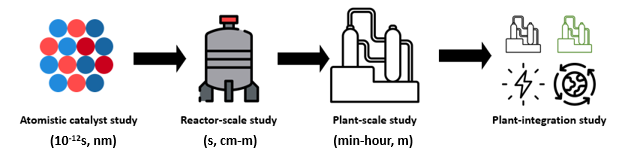
As discussed earlier, both catalyst design and reactor modelling require extremely time-consuming simulations. To attain a higher level of accuracy with reduced computational time, data-driven models like Artificial Intelligence (AI) and Machine-Learning (ML) have the potential to partially replace the existing physical models (which are computationally complex) in intensive simulations. Such replacements can bring down the computational time from several days to minutes. The advances in these fields provide us with the means to achieve multiscale simulations in a computationally efficient manner without losing accuracy. At the CCUS lab, IITM, we perform these simulations at several layers for various systems involving CO2. Carbon dioxide from the flue stack needs to be captured either at a point source or directly from the air using solid adsorbents or liquid absorbents. This process takes place in a column where CO2 comes in contact with these ab(d)sorbents. The design/simulation of these columns is not straightforward provided the complex dynamics of the process. Similarly, while utilizing the adsorbed CO2 to produce valuable chemicals, we use packed/fluidized bed catalytic reactors whose dynamics change tremendously when we move from bench-scale to larger scales. While simulating such complex scenarios, the aforementioned AI/ML tools can significantly help reduce computational time and human effort.
Authors: Ajay Koushik V, PhD, Chemical Engineering, Balivada Kusum Kumar, PhD, Chemical Engineering